10 Machine Learning ML Resume Examples - Freshers & Experts CV
8 min read
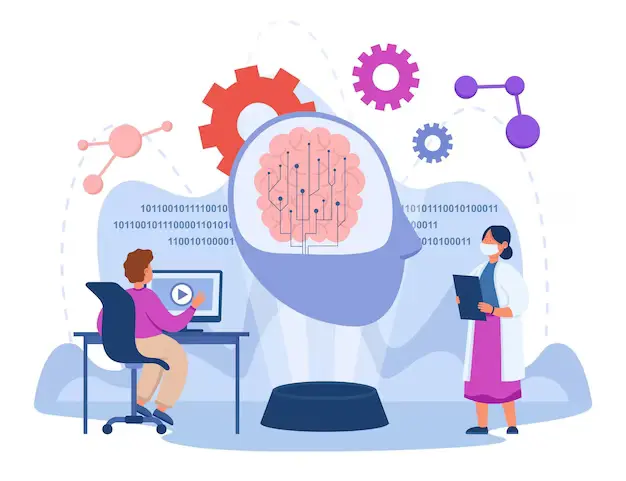
Are you building a career in Machine Learning?
If yes, then you will need a professional resume for ML along with expertise in modern machine learning algorithms to grow exponentially.
Hence, we are here for all such aspirants. Here, you will get detailed guidelines on, ‘how to craft a professional ML resume?’ and answers to additional queries including:
- What are the fundamental responsibilities of an ML engineer?
- How to become a Machine Learning Engineer in India?
- How to build a Machine Learning ML resume?
- How much do machine learning engineers earn in India?
After getting all these answers, you will need some time and a lot of focus to craft a professional machine learning resume. If you can not afford that, we recommend using any Online Resume Builder.
If not, then stick to this guide as here we will discuss about:
- Fundamental duties of a Machine Learning Engineer
- Steps to become a Machine Learning Engineer in India
- Tips to craft a professional Machine Learning Engineer resume
- Machine Learning Engineer Resume Samples
- Payscale of Machine Learning Engineers in India
Let's move on and check out all the points mentioned above in detail:
Machine Learning (ML) Engineers play a crucial role in designing, developing, and deploying machine learning models and solutions. Their responsibilities encompass a wide range of tasks related to building and maintaining ML systems. Here are the fundamental responsibilities of an ML Engineer:
- Data Collection and Preparation: Gather and preprocess data for training machine learning models. This includes data cleaning, feature engineering, and data transformation.
- Model Development: Design and build machine learning models tailored to specific tasks, such as classification, regression, clustering, or recommendation systems.
- Algorithm Selection: Choose the appropriate machine learning algorithms and frameworks based on the problem at hand and the available data.
- Model Training: Train machine learning models using labeled data, ensuring that they generalize well to make accurate predictions on new, unseen data.
- Hyperparameter Tuning: Optimize hyperparameters of machine learning models to achieve the best performance, often using techniques like grid search or Bayesian optimization.
- Feature Selection and Engineering: Identify relevant features and create new ones to improve model performance and interpretability.
- Model Evaluation: Assess the performance of ML models using appropriate evaluation metrics, cross-validation, and techniques like ROC curves and precision-recall curves.
- Model Deployment: Deploy machine learning models into production environments, making them accessible for real-time predictions. This may involve containerization and cloud services.
- Scalability and Efficiency: Ensure that ML models and systems are scalable and efficient, capable of handling large volumes of data and real-time requests.
- Monitoring and Maintenance: Implement monitoring systems to continuously evaluate model performance and address issues as they arise. Re-train models periodically to adapt to changing data.
- Security and Privacy: Implement security measures to protect sensitive data and ensure compliance with privacy regulations, such as GDPR.
- Collaboration: Collaborate with data scientists, data engineers, and domain experts to understand business requirements and integrate ML solutions into workflows.
- Documentation: Maintain detailed documentation of models, code, and processes to facilitate knowledge sharing and future development.
- Communication: Effectively communicate technical findings, challenges, and solutions to both technical and non-technical stakeholders.
- Research and Innovation: Stay up-to-date with the latest advancements in machine learning and AI, and apply innovative techniques to solve complex problems.
- Version Control: Use version control systems like Git to manage code repositories and track changes in ML models and scripts.
- Testing and Quality Assurance: Conduct rigorous testing to ensure that ML models function as intended and deliver accurate results.
- Feedback Loop: Continuously gather feedback from end-users and stakeholders to improve models and address user needs.
- Ethical Considerations: Be aware of ethical concerns related to AI and machine learning, such as bias and fairness, and take measures to address them in model development.
- Training and Mentorship: Provide guidance and mentorship to junior ML engineers or team members, helping them develop their skills.
Becoming a Machine Learning (ML) Engineer in India involves a combination of education, practical experience, and continuous learning. Here's a step-by-step guide on how to pursue a career as an ML Engineer in India:
- Bachelor's Degree: Start with a bachelor's degree in a relevant field such as computer science, mathematics, statistics, or engineering. This provides a strong foundation in programming, data analysis, and mathematics.
- Master's Degree (Optional): While not mandatory, pursuing a master's degree in machine learning, artificial intelligence, or data science can be beneficial. It allows for deeper specialization and access to research opportunities.
- Learn Programming Languages: Develop proficiency in programming languages commonly used in machine learning, such as Python and R. Python, in particular, is widely used in the ML community due to its rich libraries and frameworks.
- Mathematics and Statistics: Build a strong understanding of mathematics and statistics, including linear algebra, calculus, probability, and hypothesis testing. These are fundamental to ML algorithms and concepts.
- Online Courses and Tutorials: Enroll in online courses and tutorials to learn ML concepts and tools. Platforms like Coursera, edX, Udacity, and Khan Academy offer ML courses, including the popular "Machine Learning" course by Andrew Ng.
- Hands-on Experience: Practice by working on real-world ML projects and datasets. Start with simple projects and gradually tackle more complex problems. GitHub is a valuable platform for sharing your projects and code.
- Machine Learning Frameworks and Libraries: Become proficient in popular ML frameworks and libraries such as TensorFlow, PyTorch, and Keras. These tools simplify the implementation of ML models.
- Data Handling and Preprocessing: Learn how to handle and preprocess data effectively, including data cleaning, feature engineering, and data visualization. These skills are essential for working with real-world datasets.
- Deep Learning: Explore deep learning concepts, including neural networks and convolutional neural networks (CNNs), which are used in tasks like image recognition. Familiarize yourself with deep learning libraries like TensorFlow and PyTorch.
- Natural Language Processing (NLP) and Computer Vision (CV): Specialize in areas like NLP and CV, as they have a high demand in various industries. Study relevant libraries and techniques for tasks like sentiment analysis, language translation, and image classification.
- Projects and Portfolios: Build a portfolio of ML projects that showcase your skills and accomplishments. Share these projects on platforms like GitHub and LinkedIn to demonstrate your expertise to potential employers.
- Online Communities and Forums: Join online ML communities and forums, such as Kaggle, Stack Overflow, and Reddit's Machine Learning subreddit. Participating in these communities can help you learn from others and stay updated on industry trends.
- Certifications: Consider earning certifications in machine learning and data science from reputable organizations or platforms. Certifications can validate your skills and make you more marketable.
- Networking: Attend conferences, meetups, and workshops related to machine learning and AI. Networking can lead to job opportunities and collaborations with experts in the field.
- Job Search: Start your job search by applying to entry-level ML positions, such as data analyst or junior ML engineer roles. Gain practical experience while working with experienced professionals.
- Continuous Learning: Stay up-to-date with the latest advancements in machine learning by reading research papers, following blogs, and participating in online courses. Machine learning is a rapidly evolving field, and continuous learning is essential.
- Soft Skills: Develop soft skills such as problem-solving, critical thinking, and effective communication. These skills are essential for collaborating with interdisciplinary teams and presenting your findings.
Building a comprehensive Machine Learning (ML) resume requires careful attention to detail and a clear presentation of your skills, experience, and accomplishments in the field. Here's a detailed, step-by-step guide on how to build an effective ML resume:
Start with your full name at the top of the page, using a larger font size to make it stand out. Include a professional email address and a phone number that employers can use to reach you. Optionally, you can add links to your LinkedIn profile, GitHub, or personal website (if relevant and professional).
Begin with a well-crafted summary or objective statement. In 2-3 sentences, introduce yourself, emphasize your ML expertise, mention your career goals, and briefly describe the value you bring to potential employers. Tailor this section to the specific job you're applying for.
Create a dedicated skills section where you list your ML-related technical and soft skills. This section should serve as a quick reference for employers to gauge your qualifications.
Include programming languages (e.g., Python, R, Java), ML libraries and frameworks (e.g., TensorFlow, scikit-learn, PyTorch), data manipulation tools (e.g., pandas), data visualization tools (e.g. Matplotlib, Seaborn), and any other relevant skills such as deep learning, natural language processing (NLP), computer vision, or reinforcement learning.
Additionally, you must remember to include soft skills like problem-solving, critical thinking, teamwork, and communication.
List your educational qualifications in reverse chronological order (most recent degree first). Include the following details for each degree:
- Degree earned (e.g., Master of Science in Machine Learning).
- Name of the institution.
- Location (city and state).
- Graduation date.
- Major or field of study.
- GPA (if it's above 3.0 and relevant to the job).
Highlight your relevant work experience, including internships, co-op positions, and full-time roles. For each position, provide:
- Job title.
- Company name.
- Location.
- Employment dates (month and year).
- A detailed list of responsibilities, achievements, and projects related to ML. Use bullet points to make it easier to read.
- Quantify your achievements with numbers and percentages when possible. For example, "Improved model accuracy by 15% through feature engineering."
Create a dedicated section to showcase your ML projects. These can be personal, academic, or professional projects. For each project, provide:
- A concise project title.
- A brief description of the problem you addressed.
- Details of the ML techniques, algorithms, and tools you used.
- Results and key insights gained from the project.
- Any code repositories or links to project demos.
Include any relevant ML certifications, online courses, workshops, or MOOCs you've completed. Mention the issuing institution or platform and the completion date.
If you've contributed to ML research or published papers, mention them in a separate section. Include the title, publication date, and a summary of your contributions.
Highlight any awards, scholarships, or honors related to ML, data science, or your academic achievements.
Mention any memberships in ML-related organizations or associations, such as IEEE or ACM.
Include any languages you're proficient in, especially if relevant to the job or industry.
If you have a GitHub repository or an online portfolio showcasing your ML work, include the link.
Customize your resume for each job application by emphasizing skills and experiences that align with the specific job description.
Ensure your resume is free of errors in grammar, spelling, and punctuation. Use a clean, professional font and maintain consistent formatting throughout.
Aim to keep your resume to one page, but if you have extensive, relevant experience, it's acceptable to extend it to two pages.
Have a mentor, peer, or career advisor review your resume for feedback and suggestions.
A well-crafted ML resume should effectively communicate your qualifications, demonstrate your passion for machine learning, and align with the job you're applying for. Customization is key to increasing your chances of securing interviews and advancing your career in the ML field.
If you are a fresher or aspiring machine learning engineer, this resume sample will help you craft a job-winning machine learning engineer resume.
Sample Machine Learning Engineer Resume For Freshers:
[Your Name]
[Your Address]
[City, State, ZIP Code]
[Your Email Address]
[Your Phone Number]
[LinkedIn Profile URL (optional)]
Objective:
Dedicated and motivated recent graduate with a Bachelor's degree in Computer Science seeking an entry-level Machine Learning Engineer position to apply theoretical knowledge and develop practical skills in machine learning, deep learning, and data analysis.
Education:
Bachelor of Science in Computer Science
[University Name], [City, State]
[Month Year of Graduation]
- Relevant Coursework: Machine Learning, Deep Learning, Data Science, Algorithms, Data Structures, Statistics, Python Programming
- GPA: [Your GPA, if it's above 3.0]
Skills:
Technical Skills:
- Programming Languages: Python, Java, C++
- Machine Learning Frameworks: TensorFlow, scikit-learn, PyTorch
- Data Manipulation and Analysis: pandas, NumPy
- Data Visualization: Matplotlib, Seaborn
- Databases: SQL
- Version Control: Git
- IDEs: Jupyter Notebook, Visual Studio Code
Machine Learning Skills:
- Supervised and Unsupervised Learning
- Deep Learning: Neural Networks, CNNs, RNNs
- Natural Language Processing (NLP)
- Computer Vision
- Feature Engineering and Selection
- Model Evaluation and Hyperparameter Tuning
- Data Preprocessing and Cleaning
- Cross-Validation Techniques
Soft Skills:
- Problem Solving
- Analytical Thinking
- Teamwork and Collaboration
- Communication Skills
- Time Management
Projects:
1. House Price Prediction
- Developed a machine learning model to predict house prices using a dataset of real estate listings.
- Preprocessed data, performed feature engineering, and applied regression algorithms to achieve accurate predictions.
- Technologies Used: Python, scikit-learn, pandas, Matplotlib.
2. Sentiment Analysis with Deep Learning
- Implemented a sentiment analysis model using a deep learning architecture (LSTM) to classify movie reviews as positive or negative.
- Preprocessed text data, tokenized sentences, and created word embeddings for analysis.
- Technologies Used: Python, TensorFlow, Natural Language Toolkit (NLTK).
3. Handwritten Digit Recognition
- Built a convolutional neural network (CNN) to recognize handwritten digits from the MNIST dataset.
- Achieved high accuracy in digit recognition by optimizing model architecture and training parameters.
- Technologies Used: Python, TensorFlow, Keras.
Internship Experience:
Machine Learning Intern
[Company Name], [City, State]
[Month Year - Month Year]
- Assisted senior machine learning engineers in developing and refining ML models for data analysis and prediction.
- Conducted data preprocessing, feature extraction, and model evaluation for various projects.
- Collaborated with cross-functional teams to ensure the seamless integration of ML solutions into company products.
Certifications:
- Machine Learning Certification (Coursera)
- Data Science Specialization (edX)
- Deep Learning Specialization (Udacity)
Honors and Awards:
- Dean's List, [University Name]
- [Any other academic or relevant honors/awards]
Languages:
- Proficient in English and [Any other languages you speak, if applicable]
References:
Available upon request.
We have listed a Machine Learning Engineer Resume for an experienced professional below that will help you while building your resume in 2023:
Sample Machine Learning Engineer Resume For Experienced Professionals:
[Your Name]
[Your Address]
[City, State, ZIP Code]
[Your Email Address]
[Your Phone Number]
[LinkedIn Profile URL]
Objective:
6+ years experienced Machine Learning Engineer with a strong background in developing and deploying ML models. Seeking a challenging role to leverage my expertise in machine learning and data science to drive innovation and contribute to HBLS Technologies' success.
Technical Skills:
- Programming Languages: Python, Java, C++
- Machine Learning Frameworks: TensorFlow, scikit-learn, PyTorch
- Data Manipulation and Analysis: pandas, NumPy
- Data Visualization: Matplotlib, Seaborn
- Databases: SQL
Machine Learning Skills:
- Deep Learning: CNNs, RNNs, LSTM
- Natural Language Processing (NLP)
- Computer Vision
- Feature Engineering
- Model Evaluation and Hyperparameter Tuning
Soft Skills:
- Problem Solving
- Analytical Thinking
- Teamwork and Collaboration
- Communication Skills
Work Experience:
Machine Learning Engineer
HBLS Technologies, Bengaluru
April 2018 - Present
- Lead the development and deployment of machine learning models for various projects, resulting in improved product performance and customer satisfaction.
- Collaborate with data scientists, software engineers, and domain experts to define project goals and requirements.
- Architect and implement scalable ML pipelines for data collection, preprocessing, and model training.
- Perform data analysis, feature engineering, and model selection to optimize accuracy and performance.
- Deploy ML models into production environments, ensuring robustness and real-time capabilities.
- Continuously monitor and evaluate model performance, fine-tuning hyperparameters and enhancing algorithms.
- Mentor junior team members, providing guidance on ML best practices and conducting code reviews.
Machine Learning Engineer (Junior)
HBLS Technologies, Bengaluru
August 2016 - March 2018
- Collaborated with senior ML engineers on developing computer vision solutions for image analysis.
- Assisted in data preprocessing, feature extraction, and model training.
- Conducted research on emerging ML techniques and contributed to project innovation.
Education:
Master of Science in Computer Science
[University Name], Bengaluru
Graduated: May 2016
Bachelor of Engineering in Computer Science
[University Name], Bengaluru
Graduated: May 2014
Languages:
- Proficient in English and Hindi
References:
Available upon request.
The below-listed machine learning engineer resume sample will help all the ML experts to craft a suitable resume in this highly competitive era.
Sample Machine Learning Engineer Resume for Experts:
[Your Name]
[Your Address]
[City, State, ZIP Code]
[Your Email Address]
[Your Phone Number]
[LinkedIn Profile URL]
Summary:
Experienced Machine Learning Expert with a strong background in developing and deploying advanced machine learning models. Proven track record of delivering innovative solutions to complex problems. Seeking opportunities to lead and contribute to cutting-edge ML projects.
Technical Skills:
- Programming Languages: Python, Java, C++
- Machine Learning Frameworks: TensorFlow, scikit-learn, PyTorch
- Deep Learning: CNNs, RNNs, LSTM
- Natural Language Processing (NLP)
- Computer Vision
- Data Manipulation and Analysis: pandas, NumPy
- Data Visualization: Matplotlib, Seaborn
- Databases: SQL
- Version Control: Git
Soft Skills:
- Problem Solving
- Analytical Thinking
- Teamwork and Collaboration
- Communication Skills
- Leadership
Professional Experience:
Machine Learning Expert
HBLS Technologies, Bengaluru
[Joining Date] - Present
- Lead the development and deployment of machine learning models and solutions, resulting in significant improvements in product performance and user experience.
- Collaborate closely with cross-functional teams, including data scientists, software engineers, and domain experts, to define project objectives and requirements.
- Architect and implement scalable machine learning pipelines for data preprocessing, feature engineering, and model training.
- Apply advanced techniques in data analysis and feature selection to optimize model accuracy and robustness.
- Successfully deploy machine learning models in production environments, ensuring real-time capabilities and scalability.
- Continuously monitor and evaluate model performance, fine-tuning hyperparameters and staying updated with the latest advancements in the field.
- Mentor and provide guidance to junior machine learning engineers, fostering a culture of knowledge sharing and skill development.
Senior Machine Learning Engineer
HBLS Technologies, Bengaluru
[Joining Date] - [Leaving Date]
- Contributed to the development of machine learning solutions for various projects, with a focus on computer vision and natural language processing.
- Conducted extensive data preprocessing and feature engineering to prepare data for model training.
- Collaborated with senior team members to implement machine learning algorithms and evaluate model performance.
- Assisted in the research and experimentation of emerging ML techniques, resulting in improved project outcomes.
Education:
Master of Science in Machine Learning and Artificial Intelligence
[University Name], Bengaluru
[Graduation Date]
Bachelor of Engineering in Computer Science
[University Name], Bengaluru
[Graduation Date]
Languages:
- Proficient in English and Hindi
Certifications:
- [List any relevant certifications]
References:
Available upon request.
The salary of Machine Learning Engineers in India can vary significantly based on factors such as experience, location, skillset, and the specific company or organization. Here is a general salary range for Machine Learning Engineers in India at that time:
Entry-Level (0-2 years of experience)
The salary for entry-level Machine Learning Engineers can range from ₹4 lakh to ₹10 lakh per annum or more, depending on the location and the company. Smaller startups may offer lower salaries, while established tech companies and well-funded startups may offer higher compensation.
Mid-Level (2-5 years of experience)
Machine Learning Engineers with a few years of experience can expect salaries ranging from ₹10 lakh to ₹20 lakh per annum. Those with specialized skills or working in high-demand industries like finance, healthcare, or e-commerce may earn more.
Senior-Level (5+ years of experience)
Senior Machine Learning Engineers with over five years of experience and a strong track record in delivering impactful projects can command salaries upwards of ₹20 lakh per annum. In some cases, senior ML engineers working at top tech companies or in leadership roles may earn significantly more.
We have listed all the essential guidelines to frame an impeccable Machine Learning Engineer resume above. However, if you want to save time and effort, use HyreSnap Online Resume Builder to write your ML Engineer resume in 2023.
Its AI algorithm and professional resume templates will help you build an ML resume under 15 minutes. You can check the highlighting features of this modern resume builder below:
Below we have listed the key takeaways of this guide to help you cover everything you missed above:
- Pick a suitable resume format, i.e., chronological or combination
- Outline your resume with all the essential section headings
- Write your resume details in one-liner bullet points for better readability
- Add separate sections to list your key and technical skills
- Highlight your accomplishments and technical terms
- Quantify your achievements in the work experience section
- Proofread your resume after completing it to minimize errors
For additional resume or career-building assistance, please reach out to our experts at info@hyresnap.com.
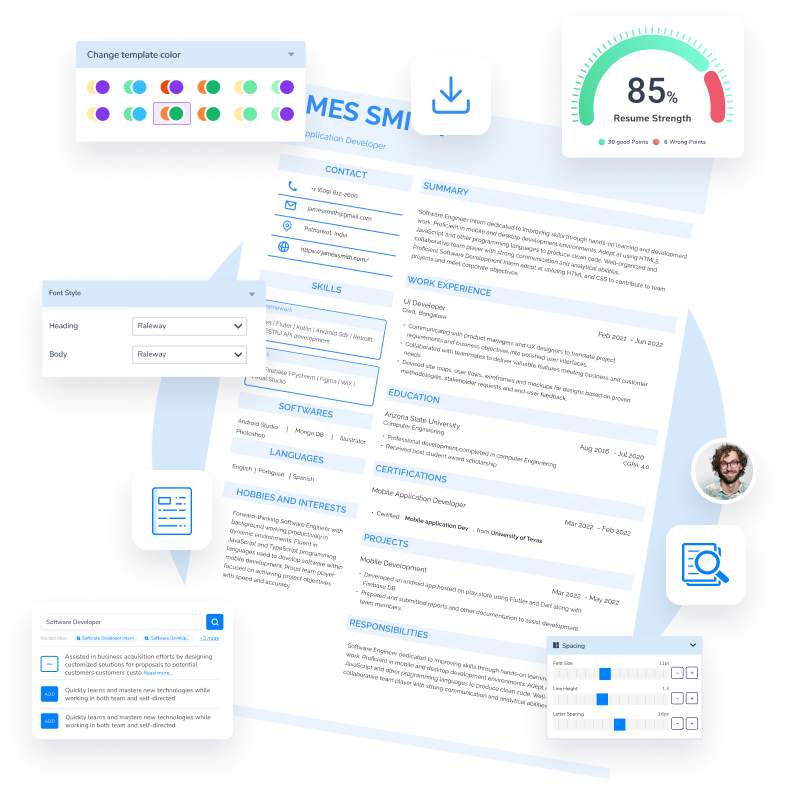
Try Now for Free!